How to optimize a QML model using Catalyst and quantum just-in-time (QJIT) compilation¶
Published: April 26, 2024. Last updated: October 7, 2024.
Note
Go to the end to download the full example code.
Once you have set up your quantum machine learning model (which typically includes deciding on your circuit architecture/ansatz, determining how you embed or integrate your data, and creating your cost function to minimize a quantity of interest), the next step is optimization. That is, setting up a classical optimization loop to find a minimal value of your cost function.
In this example, we’ll show you how to use JAX, an
autodifferentiable machine learning framework, and Optax, a
suite of JAX-compatible gradient-based optimizers, to optimize a PennyLane quantum machine learning
model which has been quantum just-in-time compiled using the qjit()
decorator and
Catalyst.
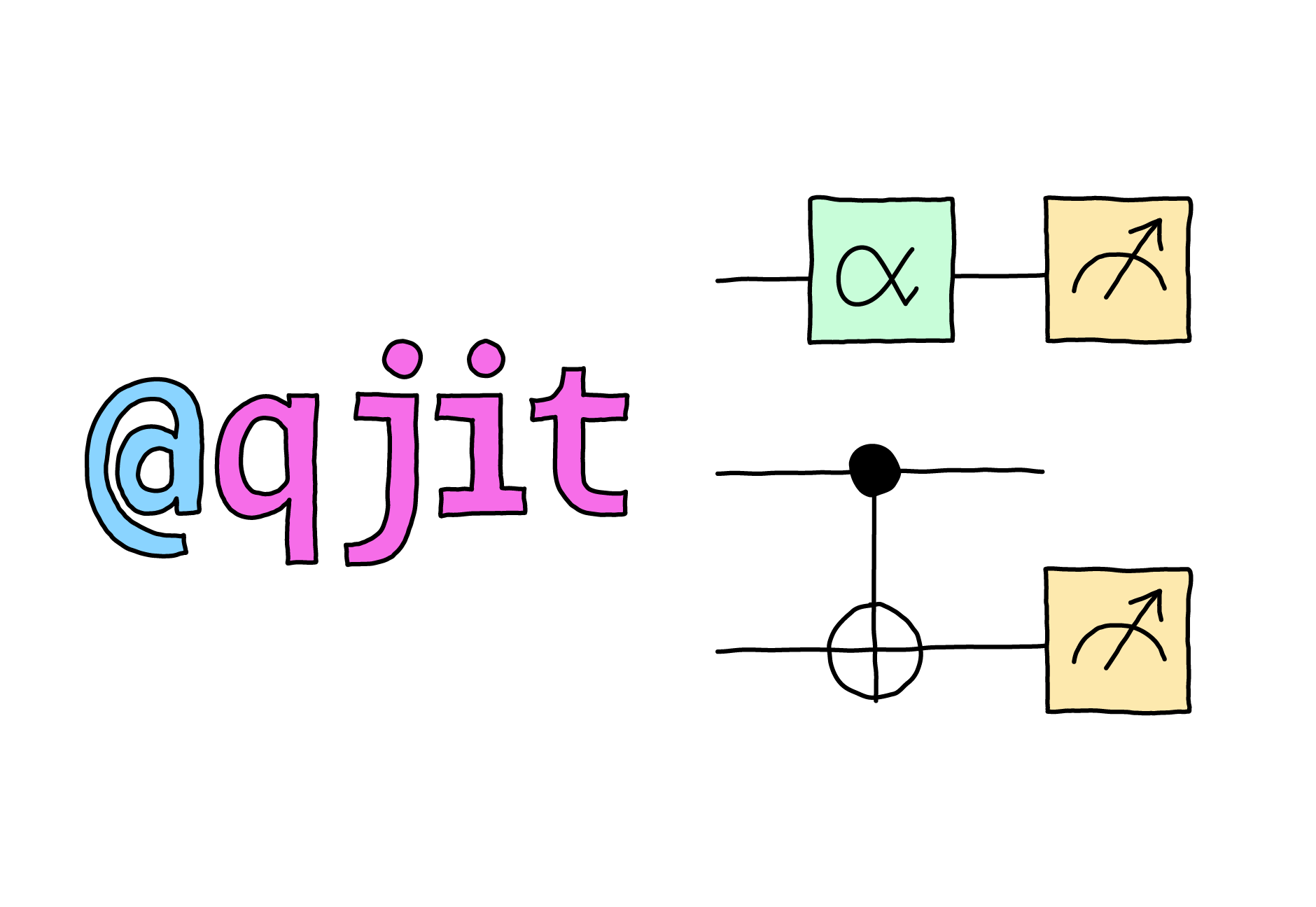
Set up your model, data, and cost¶
Here, we will create a simple QML model for our optimization. In particular:
-
We will embed our data through a series of rotation gates.
-
We will then have an ansatz of trainable rotation gates with parameters
weights
; it is these values we will train to minimize our cost function. -
We will train the QML model on
data
, a(5, 4)
array, and optimize the model to match target predictions given bytarget
.
import pennylane as qml
from jax import numpy as jnp
import optax
import catalyst
n_wires = 5
data = jnp.sin(jnp.mgrid[-2:2:0.2].reshape(n_wires, -1)) ** 3
targets = jnp.array([-0.2, 0.4, 0.35, 0.2])
dev = qml.device("lightning.qubit", wires=n_wires)
@qml.qnode(dev)
def circuit(data, weights):
"""Quantum circuit ansatz"""
@qml.for_loop(0, n_wires, 1)
def data_embedding(i):
qml.RY(data[i], wires=i)
data_embedding()
@qml.for_loop(0, n_wires, 1)
def ansatz(i):
qml.RX(weights[i, 0], wires=i)
qml.RY(weights[i, 1], wires=i)
qml.RX(weights[i, 2], wires=i)
qml.CNOT(wires=[i, (i + 1) % n_wires])
ansatz()
# we use a sum of local Z's as an observable since a
# local Z would only be affected by params on that qubit.
return qml.expval(qml.sum(*[qml.PauliZ(i) for i in range(n_wires)]))
The catalyst.vmap()
function allows us to specify that the first argument to circuit (data
)
contains a batch dimension. In this example, the batch dimension is the second axis (axis 1).
circuit = qml.qjit(catalyst.vmap(circuit, in_axes=(1, None)))
We will define a simple cost function that computes the overlap between model output and target data:
def my_model(data, weights, bias):
return circuit(data, weights) + bias
@qml.qjit
def loss_fn(params, data, targets):
predictions = my_model(data, params["weights"], params["bias"])
loss = jnp.sum((targets - predictions) ** 2 / len(data))
return loss
Note that the model above is just an example for demonstration – there are important considerations that must be taken into account when performing QML research, including methods for data embedding, circuit architecture, and cost function, in order to build models that may have use. This is still an active area of research; see our demonstrations for details.
Initialize your parameters¶
Now, we can generate our trainable parameters weights
and bias
that will be used to train
our QML model.
weights = jnp.ones([n_wires, 3])
bias = jnp.array(0.)
params = {"weights": weights, "bias": bias}
Plugging the trainable parameters, data, and target labels into our cost function, we can see the current loss as well as the parameter gradients:
loss_fn(params, data, targets)
print(qml.qjit(catalyst.grad(loss_fn, method="fd"))(params, data, targets))
{'bias': Array(-0.75432067, dtype=float64), 'weights': Array([[-1.95077271e-01, 5.28546590e-02, -4.89252073e-01],
[-1.99687794e-02, -5.32871564e-02, 9.22904864e-02],
[-2.71755507e-03, -9.64672786e-05, -4.79570827e-03],
[-6.35443870e-02, 3.61110014e-02, -2.05196876e-01],
[-9.02635405e-02, 1.63759364e-01, -5.64262612e-01]], dtype=float64)}
Create the optimizer¶
We can now use Optax to create an Adam optimizer, and train our circuit.
We first define our update_step
function, which needs to do a couple of things:
-
Compute the gradients of the loss function. We can do this via the
catalyst.grad()
function. -
Apply the update step of our optimizer via
opt.update
-
Update the parameters via
optax.apply_updates
opt = optax.adam(learning_rate=0.3)
@qml.qjit
def update_step(i, args):
params, opt_state, data, targets = args
grads = catalyst.grad(loss_fn, method="fd")(params, data, targets)
updates, opt_state = opt.update(grads, opt_state)
params = optax.apply_updates(params, updates)
return (params, opt_state, data, targets)
loss_history = []
opt_state = opt.init(params)
for i in range(100):
params, opt_state, _, _ = update_step(i, (params, opt_state, data, targets))
loss_val = loss_fn(params, data, targets)
if i % 5 == 0:
print(f"Step: {i} Loss: {loss_val}")
loss_history.append(loss_val)
Step: 0 Loss: 0.2730353743615765
Step: 5 Loss: 0.032559241075974174
Step: 10 Loss: 0.029282023100242617
Step: 15 Loss: 0.03337864978287835
Step: 20 Loss: 0.031236314161127023
Step: 25 Loss: 0.027191478076586423
Step: 30 Loss: 0.022688382016326978
Step: 35 Loss: 0.01816272636313542
Step: 40 Loss: 0.014789693503743236
Step: 45 Loss: 0.011206958477862074
Step: 50 Loss: 0.009409796244698504
Step: 55 Loss: 0.017898270572441487
Step: 60 Loss: 0.012861299047848785
Step: 65 Loss: 0.009916021521409324
Step: 70 Loss: 0.008611657371735118
Step: 75 Loss: 0.006585498631376276
Step: 80 Loss: 0.006778129326757772
Step: 85 Loss: 0.006043726885892292
Step: 90 Loss: 0.006139652588265908
Step: 95 Loss: 0.004989529670855266
JIT-compiling the optimization¶
In the above example, we just-in-time (JIT) compiled our cost function loss_fn
. However, we can
also JIT compile the entire optimization loop; this means that the for-loop around optimization is
not happening in Python, but is compiled and executed natively. This avoids (potentially costly)
data transfer between Python and our JIT compiled cost function with each update step.
params = {"weights": weights, "bias": bias}
@qml.qjit
def optimization(params, data, targets):
opt_state = opt.init(params)
args = (params, opt_state, data, targets)
(params, opt_state, _, _) = qml.for_loop(0, 100, 1)(update_step)(args)
return params
Note that we use for_loop()
rather than a standard Python for loop, to allow the control
flow to be JIT compatible.
final_params = optimization(params, data, targets)
print(final_params)
{'bias': Array(-0.75292894, dtype=float64), 'weights': Array([[ 1.63086974, 1.55018983, 0.6721261 ],
[ 0.72660604, 0.36422545, -0.75624683],
[ 2.78387503, 0.62721073, 3.44996385],
[-1.10119537, -0.12679491, 0.89283801],
[ 1.2723633 , 1.1063115 , 2.22051449]], dtype=float64)}
Timing the optimization¶
We can time the two approaches (JIT compiling just the cost function, vs JIT compiling the entire optimization loop) to explore the differences in performance:
from timeit import repeat
opt = optax.adam(learning_rate=0.3)
def optimization_noqjit(params):
opt_state = opt.init(params)
for i in range(100):
params, opt_state, _, _ = update_step(i, (params, opt_state, data, targets))
return params
reps = 5
num = 2
times = repeat("optimization_noqjit(params)", globals=globals(), number=num, repeat=reps)
result = min(times) / num
print(f"Quantum jitting just the cost (best of {reps}): {result} sec per loop")
times = repeat("optimization(params, data, targets)", globals=globals(), number=num, repeat=reps)
result = min(times) / num
print(f"Quantum jitting the entire optimization (best of {reps}): {result} sec per loop")
Quantum jitting just the cost (best of 5): 0.668785666500014 sec per loop
Quantum jitting the entire optimization (best of 5): 0.47373625000000175 sec per loop
Josh Izaac
Josh is a theoretical physicist, software tinkerer, and occasional baker. At Xanadu, he contributes to the development and growth of Xanadu’s open-source quantum software products.
Total running time of the script: (0 minutes 14.940 seconds)
Share demo